Decentralized AI: The Future of Machine Learning with BitTensor
In recent years, the field of artificial intelligence has seen tremendous growth, with centralized tech giants dominating the landscape. However, a new paradigm is emerging - decentralized AI.
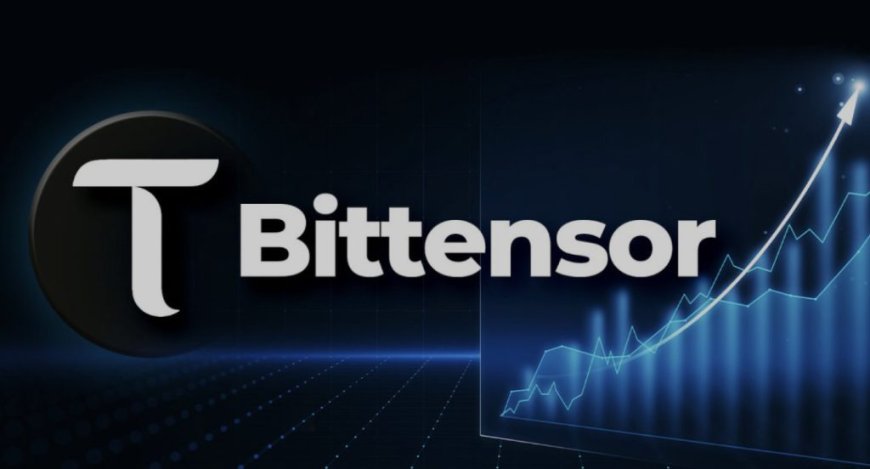
At the forefront of this revolution is BitTensor, an open-source protocol powering a decentralized machine learning network. In this article, we'll explore the intricacies of BitTensor and the potential impact of decentralized AI on the future of machine learning.
Understanding BitTensor and Decentralized AI
BitTensor aims to tackle the problem of incentivized intelligence by leveraging blockchain technology. The project's co-founder, Ala Shaabana, describes it as "incentivizing the production of machine intelligence using a blockchain." This approach combines the computational power of distributed networks like Bitcoin with the goal of advancing artificial intelligence.
The core concept behind BitTensor is creating a massive decentralized mixture of experts. In this system, individual computers run their own models, which then rank each other based on their contributions to the network as a whole. This decentralized approach aims to achieve the computational mass of networks like Bitcoin, but directed towards advancing AI rather than verifying transactions.
Key Components of the BitTensor Network
- Miners: These are the nodes in the network that perform machine learning tasks such as sentence completion, text summarization, and image generation. Miners use their computational power to execute these tasks and are rewarded with Tau tokens.
- Validators: They ensure the integrity of the network by verifying that miners are performing their assigned tasks correctly. Validators assess miners' performance over time and determine their rankings and scores.
- Tau Token: This is the cryptocurrency that powers the BitTensor ecosystem. Holding Tau tokens grants access to the network, with the amount held determining priority access.
Network Metrics and Optimizations
BitTensor employs several key metrics to maintain optimal network performance:
- Number of Peers: Currently capped at 4096, this number helps control the network's stability and allows for better testing and updates.
- Difficulty Metric: This measures the amount of work an individual miner must perform to access the network. It serves as a proof-of-work mechanism to prevent Sybil attacks and ensure miners have adequate compute power.
- Total Tau Staked: An increasing amount of staked Tau indicates growing network participation and helps maintain network honesty.
- Sequence Length: This parameter, borrowed from natural language processing, affects the quality and computational requirements of the network. Longer sequences generally result in more accurate and powerful models but require more compute power.
Challenges and Solutions in Decentralized Compute
Decentralized compute presents unique challenges compared to traditional distributed systems:
- Trust: In a decentralized system, participants are unknown to each other. BitTensor addresses this by implementing a trustless system that forces honesty between parties.
- Heterogeneous Nodes: The network must accommodate various types of participants, from research labs to individual miners. BitTensor's algorithm enables models to find peers they can learn from, regardless of architecture differences.
- Bandwidth Constraints: While high bandwidth is initially crucial, as the network grows, compute costs may overtake bandwidth as the primary bottleneck.
- Verification: To prevent collusion and cheating, BitTensor employs a system of peer ranking and validator consensus to ensure honest participation.
Security Considerations in Decentralized AI
Security is a critical aspect of any decentralized system. BitTensor faces several security challenges:
- Sybil Attacks: The difficulty metric helps prevent users from flooding the network with multiple low-quality nodes.
- Collusion: The validator system and peer ranking mechanism work to identify and discourage collusion among nodes.
- Data Quality: As the network expands to different modalities (e.g., images), ensuring the quality and appropriateness of data becomes crucial.
- Network Spamming: Measures are in place to prevent nodes from submitting garbage data to earn rewards without contributing meaningful compute.
Maximizing Network Utilization
Ensuring high utilization of all nodes is crucial for the network's efficiency. BitTensor approaches this challenge through:
- Incentivization: Nodes are rewarded based on the quality and quantity of their contributions, encouraging high utilization.
- Quality-based Routing: Higher-performing nodes with better bandwidth are more likely to receive tasks, naturally increasing their utilization.
- Adaptive Difficulty: The network adjusts its difficulty to maintain optimal participation levels.
Decentralized vs. Centralized AI Training
Training AI models on a decentralized network like BitTensor differs significantly from centralized cloud solutions:
- Inter-model Communication: During training, models in BitTensor communicate with other nodes, potentially leading to more resilient and generalist models.
- Dynamic Data Exposure: Models are exposed to a broader range of data from other nodes, potentially improving generalization.
- Peer Selection: Models learn which peers are most useful for their training, creating a form of meta-learning.
The Future of Decentralized AI
As the field of AI continues to evolve, decentralized systems like BitTensor may play an increasingly important role:
- Democratization of AI: By distributing compute and ownership, decentralized AI could prevent the monopolization of AI technology by a few large corporations.
- Diverse Perspectives: A global network of AI contributors could lead to more diverse and robust AI systems.
- Edge Computing: Future iterations might involve networks of smaller devices, bringing AI compute closer to the data source.
- Ethical Considerations: Decentralized governance models could help address some of the ethical concerns surrounding AI development.
Challenges and Misconceptions
Despite its potential, decentralized AI faces several challenges:
- Technical Complexity: Coordinating a decentralized network of AI developers and miners presents significant technical hurdles.
- Adoption Barriers: Traditional AI researchers may be skeptical of blockchain-based solutions, slowing adoption.
- Regulatory Uncertainty: As a novel approach to AI development, decentralized AI may face regulatory challenges in various jurisdictions.
- Misconceptions: The association with cryptocurrency speculation can overshadow the technological innovations in decentralized AI.
Advice for ML Developers Entering Decentralized AI
For machine learning developers interested in decentralized AI, Shaabana offers the following advice:
- Learn Distributed Computing: Understanding the basics of distributed systems is crucial for working with decentralized AI.
- Focus on Infrastructure: Decentralized AI often requires a deeper understanding of infrastructure than traditional ML development.
- Embrace Complexity: Be prepared to tackle new challenges that don't typically arise in centralized AI development.
- Stay Open-minded: The field is rapidly evolving, and new solutions to current limitations may emerge quickly.
Conclusion: The Promise of Decentralized AI
Decentralized AI, as exemplified by projects like BitTensor, represents a paradigm shift in how we approach artificial intelligence. By leveraging blockchain technology and distributed computing, these systems aim to democratize AI development, potentially leading to more robust, diverse, and ethically sound AI systems.
While challenges remain, particularly in areas of security, network coordination, and adoption, the potential benefits of decentralized AI are significant. As the technology matures, we may see a future where AI development is not dominated by a few large players but is instead a collaborative, global effort.
The success of decentralized AI will depend on continued innovation in areas such as distributed computing, blockchain technology, and machine learning algorithms. It will also require a shift in mindset among AI researchers and developers, embracing the potential of decentralized systems.
As we move forward, projects like BitTensor serve as important experiments in this new paradigm. They challenge our assumptions about how AI should be developed and deployed, potentially paving the way for a more open, transparent, and democratically governed AI ecosystem.
The journey of decentralized AI is just beginning, and its full impact remains to be seen. However, as the field continues to evolve, it offers an exciting alternative to the current centralized model of AI development. By distributing both the computational work and the governance of AI systems, decentralized AI could help ensure that the benefits of this powerful technology are shared more widely and equitably across society.
Source : @The Bittensor Hub.