Streamlit Multimodal Llama 3.1 and Subnet 24: Revolutionizing AI With Omega Focus and Beyond
In the world of AI and machine learning, progress is moving at a rapid pace, with multimodal models and decentralized systems reshaping how data is processed and utilized. Recently, there have been some exciting developments in AI that focus on improving caption quality, creating decentralized data marketplaces, and enhancing user productivity.
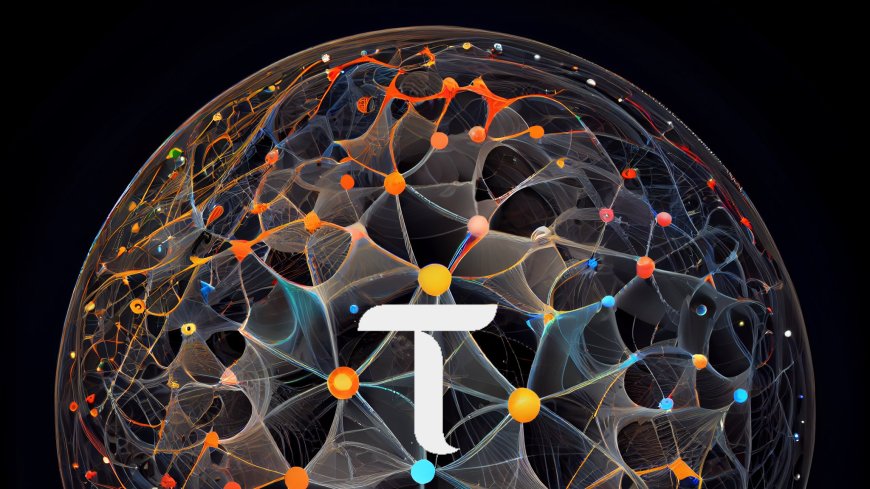
Introduction
In the world of AI and machine learning, progress is moving at a rapid pace, with multimodal models and decentralized systems reshaping how data is processed and utilized. Recently, there have been some exciting developments in AI that focus on improving caption quality, creating decentralized data marketplaces, and enhancing user productivity. This article dives deep into the innovations surrounding Streamlit multimodal Llama 3.1, Subnet 24, and Omega Focus, which are setting new standards for AI-driven systems. With advancements in caption scoring, focus management, and the utilization of real-world data, the future of AI looks more promising than ever.
Enhancing Caption Quality with Subnet 24
One of the most significant updates involves improving the quality of captions within Subnet 24, a platform designed to handle large amounts of data across multiple modalities, including text, video, and audio. Initially, the platform relied on ImageBind, a tool trained on YouTube videos and their corresponding titles. However, ImageBind had some limitations, as it favored simpler titles over more detailed descriptions. This led to a situation where accurate, descriptive captions were penalized compared to basic titles.
To address this issue, the team expanded the context window of ImageBind, allowing for more comprehensive representations of the data. The result is a scoring system that better evaluates videos, audio, and text, rewarding high-quality captions instead of penalizing them.
Example of Caption Scoring Improvement
Before the update, two captions for the same video were scored as follows: a simple title received a score of 0.33, while a detailed description earned a much lower 0.11. With the new system in place, the title score dropped to 0.24, and the detailed description improved to 0.36. This reflects a significant improvement in how well the system evaluates and rewards more descriptive and accurate captions.
In addition to refining the context, the team introduced new heuristics, such as evaluating the length and uniqueness of the words in the description. By doing so, the system can further distinguish between low-effort captions and more detailed, higher-quality ones. For instance, after applying these heuristics, the title score dropped further to -0.02, while the detailed caption maintained a 0.2 score.
Tackling Caption Exploitation with the Critic Model
Another challenge in improving caption quality is the ongoing "cat-and-mouse game" between the platform and users trying to exploit the system. Some users generate random captions to manipulate the similarity algorithms and gain higher scores. In response, the team developed a new critic model that uses a combination of different scores, such as similarity, audio-text alignment, and description length. These scores are aggregated to create a more balanced evaluation, rewarding well-detailed captions and penalizing low-effort ones.
The critic model has played a crucial role in enhancing the quality of data submitted to Subnet 24. By programmatically improving caption scoring, the platform ensures that miners—those who contribute and validate data—cannot game the system as easily. Regular model retraining helps the platform stay ahead of exploitation attempts, ensuring the integrity and accuracy of the data being processed.
Omega Focus: Revolutionizing Productivity and Data Collection
Moving beyond caption quality, the introduction of Omega Focus represents a major breakthrough in AI-driven productivity tools. Omega Focus is designed to monitor user focus, record screen activity, and incentivize the submission of screen recordings to Subnet 24. The tool analyzes sequential reasoning steps to help tackle one of the common issues in AI—hallucination—which occurs when AI models produce incorrect or irrelevant information.
How Omega Focus Works
Omega Focus integrates with various tools such as Discord, Twitter, Notion, and Obsidian, aiming to expand into other services. The platform functions as a flow state machine that enhances user productivity by guiding tasks based on context from tools like Google Calendar, Notion, and Twitter. For instance, if a user is working on a task related to Omega Labs’ planning and becomes distracted, Omega Focus sends a fun notification—perhaps featuring a humorous character like Trump—reminding the user to stay on track. Meanwhile, the system records the user’s screen activity to ensure it aligns with the intended task.
Once a session is recorded, the system processes it, and validators score it based on productivity. Users can then submit the recordings to the decentralized data marketplace, where miners purchase them based on the AI-assigned reward score.
Building a Marketplace for Screen Recordings
Omega Focus creates a unique marketplace for screen recordings, where users are rewarded for their attention and focus. This marketplace bridges the gap between productivity and monetization, allowing users to earn rewards by contributing valuable data. Once a recording is purchased by a miner, it undergoes validation, and miners receive emissions—rewards distributed over time based on the value of the data.
The system is designed to ensure that miners generally break even or profit from their activities. However, due to the dynamic nature of emissions, there are periods when miners may experience fluctuations in earnings. This iterative process aims to continuously improve the design and functionality of the marketplace, making it a robust ecosystem for data exchange.
Why Miners Are Essential
A key question raised during the development of Omega Focus is why the system relies on miners to purchase recordings instead of Omega buying them directly. The decentralized approach allows for greater validation and contribution from diverse sources, ensuring the robustness of the data. Miners play a critical role in this process, contributing valuable real-world data points from a variety of activities. This approach promotes a more secure and decentralized ecosystem, ensuring that the system is not reliant on a single entity for data validation.
Subnet 21 and 24: Pushing the Boundaries of Multimodal AI
The conversation around Subnet 24 naturally extends to Subnet 21, where the goal is to develop an omni-model capable of understanding and generating across multiple modalities—image, audio, and video. Subnet 21 is making significant strides in understanding these modalities, with plans to integrate more sophisticated evaluation mechanisms for tasks such as video generation.
The team is working on expanding the types of models accepted into the system and providing training scripts to miners, allowing for better model submissions. By building a decentralized, multimodal AI system, the team aims to surpass traditional benchmarks and create a system where users and miners can earn rewards from a wide range of contributions, from basic tasks to advanced modeling.
Conclusion: A Decentralized Future for AI
The developments discussed above—improving caption quality, launching Omega Focus, and advancing Subnet 21 and Subnet 24—represent major strides in the field of AI. The emphasis on decentralization, user contribution, and the use of real-world data sets a new precedent for how AI systems can operate and evolve. As AI models become more capable of understanding and generating across different modalities, the potential for automating workflows and innovating in various industries grows exponentially.
With Streamlit multimodal Llama 3.1, Subnet 24, and Omega Focus leading the charge, the future of AI looks increasingly decentralized, user-focused, and productive. The possibilities for innovation are vast, and the next steps in this journey will undoubtedly push the boundaries of what AI can achieve.
Source : @Opentensor Foundation