Exploring the Future of Decentralized Neural Networks and Time Series Data
In a world where data has become the lifeblood of decision-making, decentralized systems offer a promising avenue for handling various types of data streams. Today, we're diving deep into the exciting developments around time series subnetworks and data streams, focusing on their transformative potential across industries
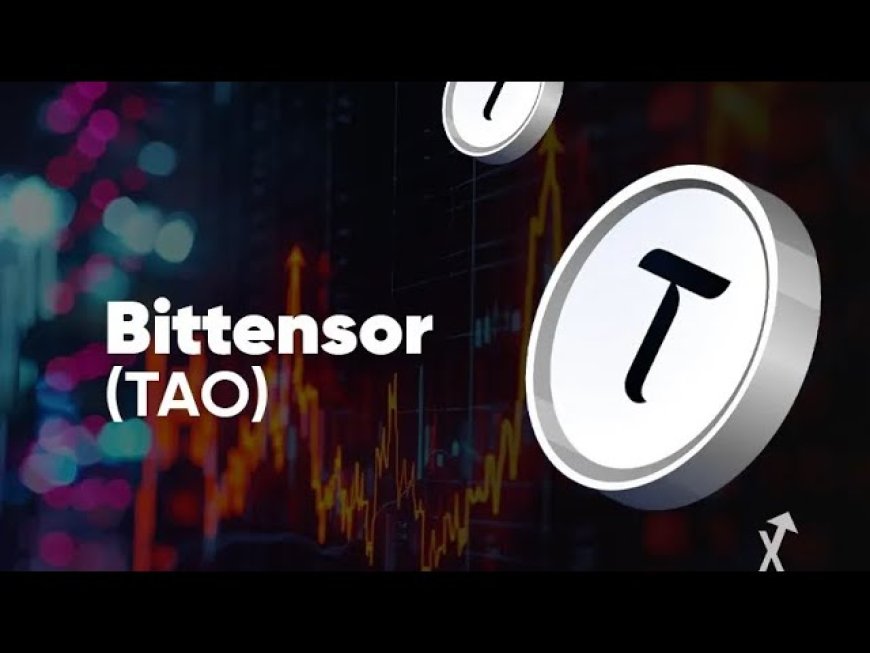
Introduction
In a world where data has become the lifeblood of decision-making, decentralized systems offer a promising avenue for handling various types of data streams. Today, we're diving deep into the exciting developments around time series subnetworks and data streams, focusing on their transformative potential across industries. Our guest, Arash, is pushing the envelope by developing a subnetwork that goes beyond just one type of input, capable of supporting continuous data and stream data, making the system incredibly versatile.
This article explores how this innovation could change the landscape of data processing, the role of validators, the challenges involved in collusion detection, and the immense possibilities that arise when combining neural networks with predictive applications.
A Revolutionary Time Series Subnetwork
Arash has been spearheading a new approach to time series subnetworks, which initially began as a tool for algorithmic trading. The aim was simple: create a marketplace of competing neural nets to generate the best trading algorithms. However, the idea soon evolved into something broader—stream data. This allows the subnetwork to handle a vast array of datasets, from stock predictions to anomaly detection, applicable to SaaS companies and beyond.
The beauty of this system lies in its flexibility. Validators are no longer bound to specific data types such as stocks; they can work with any dataset. This opens a world of possibilities for applications, ranging from sports gambling predictions to supply chain analytics. Clients can define topics within the subnetwork, train miners on their data, and even build decentralized prediction models in sectors that extend far beyond finance.
Scalable Cost-Sharing for Validators
One of the major innovations in this new subnetwork is a scalable cost-sharing platform for validators. This allows them to share or sell unused request capacity to others, irrespective of the subnetwork they are on. It creates a highly efficient ecosystem where validators can optimize resources, contributing to a more sustainable and flexible network.
The potential here is huge. Imagine a network that allows multiple sectors—from finance to SaaS analytics—to plug into a system that can train its miners on specialized data sets, ultimately resulting in a highly accurate, decentralized prediction system. The collaborative aspect of this model is key to its success, enabling clients and miners to work together to create better, more efficient algorithms.
Decentralized Algorithms and Their Broad Applications
This approach could revolutionize sectors that rely heavily on proprietary algorithms, like stock predictions. Instead of closed systems where big players like Bloomberg or BlackRock dominate, the network will offer a decentralized alternative where the best algorithms rise to the top, thanks to a marketplace of competing neural networks.
For example, a time series subnetwork doesn’t just forecast stock prices. It can be trained to generate strategies on how to use those predictions, providing actionable insights rather than just raw data. This has profound implications for industries such as sports gambling, where accurate predictions could lead to significant advantages.
Anomaly detection is another area with vast potential. This technology can be applied to supply chains, SaaS companies, and even sports data. It allows for the building of comprehensive analytics systems that can identify irregularities or patterns, improving efficiency and reducing risks.
Collusion Detection: A Key Challenge
With such an expansive system, preventing collusion between miners and validators is a significant challenge. Arash's team is working hard to implement mechanisms that will detect and deter collusion, ensuring that miners and validators act in good faith. The rules around data integrity and healthy collaboration are essential to maintaining a transparent and fair network.
The reward system plays a crucial role in preventing bad actors from gaming the system. Miners are incentivized to stay in the network because leaving would allow others to improve upon their work and earn more rewards. This dynamic creates a competitive but fair environment where the focus remains on producing the best possible results.
The Future of Time Series Data
The future of time series data and decentralized networks is incredibly promising. While initial adoption may be slow, especially during the early stages, the community's interest in these technologies is growing. As more miners become specialized and clients provide input, the marketplace will naturally evolve, delivering better results over time.
For instance, stock prediction models within the network are already showing potential. Instead of using isolated algorithms, the collaborative nature of the system will enable it to produce more accurate, up-to-date predictions. This could even lead to sector-specific solutions, where neural networks are trained for specific tasks like consulting or analytics.
The potential applications go beyond trading and gambling. Consulting businesses and other industry-specific models will find significant value in this technology, thanks to its scalability and adaptability. The ability to generalize stream data, rather than limiting it to just one type of input, makes this subnetwork a groundbreaking development in AI.
Combining Time Series Data with Large Language Models (LLMs)
One of the most exciting possibilities lies in combining time series data with large language models (LLMs). As Arash mentioned, text data from various media sources—like articles or trading blogs—can be converted into numerical data and integrated into hybrid predictive models. This opens up an entirely new frontier in predictive applications, where language models and time series data work together to enhance predictions.
For example, converting text into floating points could allow a system to process news articles or blog posts related to stock movements, enabling a more comprehensive and dynamic stock prediction model. This combination of textual and numerical data offers endless possibilities for AI development, and it's an area that the team is actively exploring.
The Road Ahead: Decentralization and Community Ownership
As the network grows, Arash’s team aims to transition from a centralized to a fully decentralized system. In the long term, this decentralized structure will allow anyone to run a request layer, enabling broader participation and further enhancing data integrity.
The ultimate goal is to build a community-owned network where clients collaborate to achieve the best results. Over time, as more people join the ecosystem, it will improve faster, leading to even better predictive models and more accurate neural networks.
Conclusion
The future of decentralized time series data and neural networks is bright. The innovations happening now in stream data and validators are just the beginning. The possibilities for predictive models across industries are immense, and as Arash’s team continues to refine the system, we can expect even more groundbreaking developments in the near future.
This ecosystem will thrive on collaboration, competition, and continuous improvement, pushing the boundaries of what’s possible in the realm of decentralized AI.