Liquid Alpha: Revolutionizing Bittensor's Consensus Mechanism to Combat Weight Copying
In a groundbreaking development for the Bittensor network, the Open Tensor Foundation has unveiled Liquid Alpha, a revolutionary upgrade to the consensus mechanism designed to combat weight copying and enhance the overall efficiency of the network. This article delves into the intricacies of Liquid Alpha, its implementation, and its potential impact on the Bittensor ecosystem.
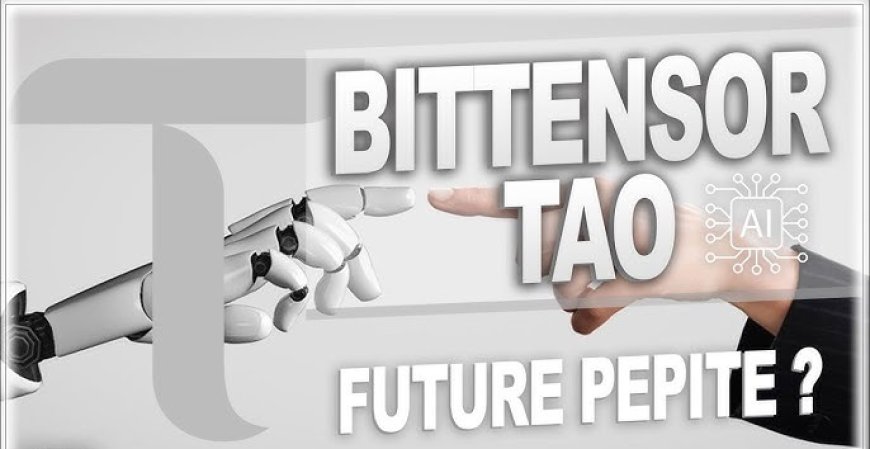
Understanding Liquid Alpha
Liquid Alpha is a sophisticated extension of Bittensor's existing consensus algorithm, aimed at penalizing weight copiers and incentivizing honest validation. At its core, Liquid Alpha introduces a dynamic element to the bond accumulation process, making it more speculative and time-sensitive.
The key innovation lies in the modification of the bonds matrix, which determines validator dividends. With Liquid Alpha, the rate at which validators acquire ownership (bonds) in miners becomes adaptive, depending on the consensus level of each miner. This change forces validators to identify miners that will eventually reach consensus, rather than those currently in consensus.
Mathematical Foundation
The Liquid Alpha mechanism is based on a complex mathematical model, detailed in a research paper by the Open Tensor Foundation's Pineal team. The model represents validator interactions as a repeated game, where players (validators) can observe and potentially copy actions from previous rounds.
The core of the change is expressed in Equation 17 of the paper, which describes how the bonds matrix evolves over time based on a moving average. This evolution is governed by an adaptive alpha parameter, calculated using a sigmoid function (Equation 30) that maps consensus to alpha values.
The mathematical model takes into account several key factors:
- The set of validators and miners
- A sequence of rounds in which actions are taken
- Signals observed by validators about miner performance
- Weight vectors reported by validators
- The calculation of consensus values for each miner
- The evolution of bonds over time
This comprehensive model allows for a nuanced understanding of the interactions between validators and miners, providing a solid foundation for the implementation of Liquid Alpha.
Implementation and Tuning
Implementing Liquid Alpha requires careful tuning of hyperparameters, specifically the alpha_low and alpha_high values, as well as the commit-reveal interval. The Open Tensor Foundation has provided a Jupyter notebook that subnet owners can use to determine optimal settings for their specific subnet.
The notebook performs a backtest on historical subnet data, evaluating different hyperparameter combinations to maximize the penalty on weight copiers while minimizing impact on honest validators. The process involves:
- Collecting historical data (approximately 3 days' worth)
- Running simulations with various parameter combinations
- Analyzing results to identify optimal settings
- Verifying the impact on honest validators and weight copiers
The tuning process is critical for the effective implementation of Liquid Alpha. Subnet owners must consider the unique characteristics of their subnet, including:
- The frequency and magnitude of weight changes
- The typical consensus patterns among miners
- The existing level of weight copying in the subnet
- The desired balance between penalizing weight copiers and maintaining incentives for honest validators
By carefully analyzing these factors and using the provided tools, subnet owners can optimize Liquid Alpha for their specific use case.
Benefits and Challenges
Liquid Alpha, when properly implemented, can significantly reduce the profitability of weight copying. Simulations have shown that it can potentially reduce weight copier dividends by up to 8% compared to honest validators. This substantial difference creates a strong incentive for validators to engage in genuine evaluation rather than simply copying weights.
The benefits of Liquid Alpha extend beyond just penalizing weight copiers. By encouraging more active participation from validators, it can lead to:
- Faster consensus finding on high-quality miners
- More dynamic and responsive subnets
- Improved overall network performance
- A more competitive and engaging environment for validators
However, the effectiveness of Liquid Alpha depends on several factors:
- The dynamism of the subnet's incentive landscape
- The magnitude of weight changes within the subnet
- Proper tuning of hyperparameters
- Implementation in conjunction with the commit-reveal mechanism
It's important to note that Liquid Alpha is not a one-size-fits-all solution. Its effectiveness may vary depending on the specific characteristics of each subnet. Some subnets may see dramatic improvements, while others might experience more subtle changes.
Challenges in implementing Liquid Alpha include:
- The complexity of the mechanism, which may be daunting for new subnet owners
- The need for ongoing monitoring and potential re-tuning as subnet dynamics change
- Ensuring that the implementation doesn't inadvertently disadvantage honest validators
- Educating the broader Bittensor community about the benefits and proper use of Liquid Alpha
Implications for Subnet Owners
For subnet owners, implementing Liquid Alpha represents both an opportunity and a challenge. While it offers a powerful tool to combat weight copying, it also requires a deep understanding of the subnet's dynamics and careful parameter tuning.
The Open Tensor Foundation recommends that subnet owners:
- Familiarize themselves with the Liquid Alpha concept and mathematical model
- Use the provided Jupyter notebook to analyze their subnet and determine optimal parameters
- Implement Liquid Alpha in conjunction with commit-reveal for maximum effectiveness
- Monitor the impact on validator behavior and subnet performance
- Be prepared to adjust parameters as the subnet evolves
Subnet owners should also consider the following when implementing Liquid Alpha:
- The current state of their subnet: Is weight copying a significant issue? How dynamic is the incentive landscape?
- The goals for their subnet: Are they aiming for rapid consensus, high stability, or a balance of both?
- The technical capabilities of their team: Do they have the expertise to implement and manage Liquid Alpha effectively?
- The potential impact on existing validators: How will the change affect current participants in the subnet?
By carefully considering these factors, subnet owners can make informed decisions about implementing Liquid Alpha and maximize its benefits for their subnet.
Future Developments
The introduction of Liquid Alpha is part of a broader strategy to enhance Bittensor's consensus mechanism and promote decentralization. Future developments include:
- Child hot keys: A mechanism to distribute the validation load across multiple keys, addressing the scalability challenges faced by validators managing multiple subnets. This development is particularly exciting as it could dramatically change the way validators operate within the Bittensor network.
- Reduced minimum take: Plans to lower the minimum take from 9%, potentially in conjunction with the introduction of child keys. This change could make it more attractive for validators to specialize in specific subnets, further enhancing the network's efficiency.
- Ongoing research: Continued refinement of the consensus mechanism based on real-world performance data and community feedback. The Open Tensor Foundation is committed to iterative improvement, ensuring that Bittensor remains at the cutting edge of decentralized machine learning technology.
- Enhanced educational resources: Recognizing the complexity of these new mechanisms, the foundation plans to develop more comprehensive guides, tutorials, and tools to help subnet owners and validators understand and implement these advanced features.
- Improved monitoring and analytics tools: To help subnet owners and validators track the impact of Liquid Alpha and other innovations, the foundation is working on developing more sophisticated monitoring and analytics tools.
Community Engagement and Feedback
The success of Liquid Alpha and similar innovations heavily relies on active community engagement and feedback. The Open Tensor Foundation encourages all members of the Bittensor community to:
- Experiment with Liquid Alpha on test networks
- Share experiences and insights in community forums
- Provide feedback on the implementation process and results
- Suggest improvements or additional features
- Collaborate on developing best practices for using Liquid Alpha
This collaborative approach ensures that the development of Bittensor remains aligned with the needs and experiences of its users, fostering a truly decentralized and community-driven ecosystem.
Conclusion
Liquid Alpha represents a significant leap forward in Bittensor's ongoing efforts to create a robust, efficient, and fair network for decentralized machine learning. By introducing a more dynamic and speculative element to the consensus mechanism, it challenges validators to engage more actively in the evaluation process, potentially leading to faster consensus finding and improved overall network performance.
While the implementation of Liquid Alpha may seem complex, particularly for new subnet owners, it offers a powerful tool for those looking to optimize their subnet's performance and combat weight copying. As the Bittensor ecosystem continues to evolve, tools like Liquid Alpha will play a crucial role in shaping its future.
The Open Tensor Foundation encourages all members of the Bittensor community, especially subnet owners and power users, to dive deep into the concepts behind Liquid Alpha. By understanding and leveraging this new mechanism, the community can collectively work towards a more efficient, decentralized, and robust network.
As we move forward, the success of Liquid Alpha and similar innovations will depend on the active participation and feedback from the community. The Open Tensor Foundation remains committed to providing support, documentation, and ongoing improvements to ensure that Bittensor remains at the forefront of decentralized machine learning technology.
The introduction of Liquid Alpha marks an exciting new chapter in the Bittensor story. It demonstrates the network's commitment to continuous improvement and its ability to address complex challenges in novel ways. As the technology matures and more subnet owners implement Liquid Alpha, we can expect to see a more dynamic, efficient, and resilient Bittensor network emerge.
For those interested in learning more about Liquid Alpha or getting involved in its implementation, the Open Tensor Foundation provides extensive resources, including detailed documentation, code repositories, and community forums. By leveraging these resources and actively participating in the Bittensor ecosystem, individuals and organizations can play a crucial role in shaping the future of decentralized machine learning.
Source : @Opentensor Foundation