Building the World’s Largest Open-Source Multimodal Dataset: Exploring Subnet 24 Omega on BitTensor
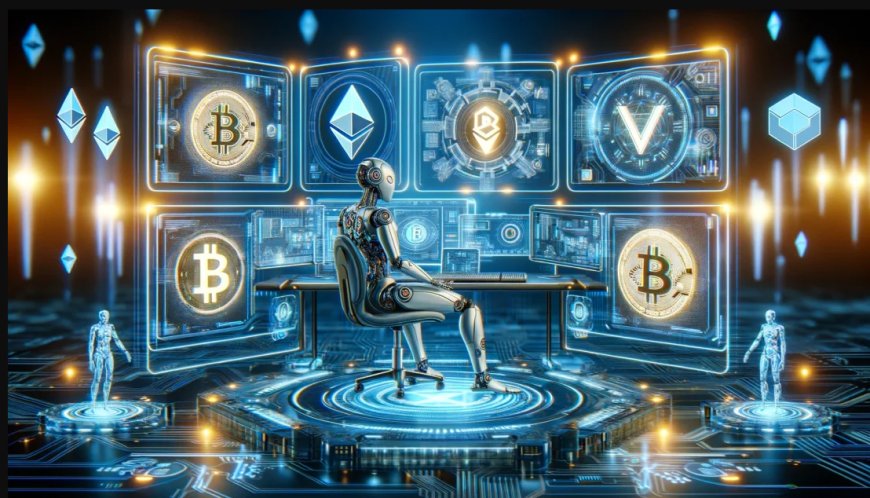
What is Subnet 24 Omega?
At its core, Subnet 24 Omega is focused on the collection and refinement of a multimodal dataset, where multiple types of data—such as video, audio, and text—are aligned in the same dataset rows. This enables AI models to understand relationships across different types of content, opening the door to advanced generative and relational capabilities.
Unlike traditional datasets that focus on one modality, such as text or images, a multimodal dataset allows AI to learn from the relationships between these modalities. For example, video content is paired with accurate captions and audio data, giving AI models a richer context to learn from. This type of data is invaluable for Artificial General Intelligence (AGI), the next frontier in AI research, where models need to generate diverse outputs from various inputs.
Omega Labs, a team previously behind Wombo, is pioneering this effort. They have spent years working at the intersection of AI and creative entertainment, developing apps like Wombo's selfie-singing tool and the Dream app for art generation. Their work in this space highlighted the enormous potential of multimodal data, which led them to form Omega Labs with the goal of creating an open-source dataset of unprecedented size and quality.
The Role of BitTensor
An essential component of Subnet 24 Omega is the use of BitTensor, a decentralized machine learning protocol that allows for collaborative AI training. BitTensor's incentivization system plays a critical role in gathering and curating the dataset. Miners, incentivized through the BitTensor network, contribute videos with accurate captions to enhance the dataset's quality. This decentralized approach ensures a more diverse and continuously evolving dataset.
Incentivizing miners to contribute data ensures that the project scales rapidly while maintaining quality. Miners are rewarded based on both the quantity of data they provide and, more importantly, the relevance and quality of the captions they generate. High-quality captions improve the training efficiency and performance of AI models, as evidenced by the progression from open models like GPT-2 to GPT-3, where detailed captions significantly boosted model performance.
Multimodal Data and AI Training
One of the primary challenges in AI today is the need for high-quality multimodal data to train advanced models. Subnet 24 Omega addresses this challenge by building a dataset that aligns various modalities—such as video, audio, and text—within a unified semantic space. The project leverages cutting-edge models like Meta's ImageBind, which enables multimodal search capabilities by embedding these different data types into the same semantic space.
The goal is to surpass existing datasets like Intern Video, which consists of 25 to 30 million videos but with relatively simple captions. Subnet 24 Omega, with its 16 million captioned YouTube videos and growing at a rate of 500,000 new videos per day, aims to not only match but exceed the size and quality of these state-of-the-art datasets. By enriching videos with detailed captions, Omega Labs ensures that the data is valuable for training sophisticated AI systems.
Detailed captions do more than just describe videos; they drastically reduce the compute requirements for training. As seen in the PixArt 2 paper, detailed captioning reduces the compute costs by about five times, making it a cost-efficient solution for large-scale AI training. This reduction in compute costs, combined with the ability to generate complex captions, makes Subnet 24 Omega's dataset a game-changer for any-to-any models—models that can take any input and generate any output.
The Impact of Subnet 24 Omega on AI Research
The impact of Subnet 24 Omega on AI research could be profound. With a multimodal dataset, models like Sora and Stability AI can learn to generate more realistic and relational content across different data types. Imagine AI models that can take a video, analyze its audio, and generate text summaries, or models that can create video content from audio cues—all made possible by the integration of multimodal datasets.
By building on the foundation of multimodal AI research, Omega Labs is unlocking new possibilities for generative models. These models, when trained on rich, interconnected datasets, can learn to perform complex tasks such as zero-shot generation of multimodal content, where the model creates audio, video, and text simultaneously without prior training on specific examples.
The potential applications of these advancements are limitless. For instance, industries like entertainment, education, and healthcare could all benefit from AI systems that can generate and understand multimodal content. AI could generate training videos for medical professionals by analyzing and synthesizing video, audio, and text data. Similarly, AI-generated educational content could be made more interactive and engaging by integrating multiple data types.
Challenges and Future Outlook
While the potential is immense, the challenges associated with building and maintaining such a large dataset are significant. One major challenge is ensuring data quality at scale. Although miners are incentivized to submit high-quality captions, there are risks related to centralization and overfitting. For example, miners might submit duplicate data or videos with similar captions, reducing the dataset's diversity.
To address this, Omega Labs is developing strategies to assess the quality of the dataset continuously. This includes running similarity models like ImageBind to calculate the relevance of text captions to videos and using human annotators when necessary to validate the quality of the data.
As the system evolves, there are also plans to expand the scope of the dataset beyond video, audio, and text. Emerging modalities such as brain wave data could be integrated into the dataset, enabling even more advanced AI applications. However, with these advancements come new challenges related to data safety, privacy, and ethical considerations.
Monetization and Sustainability
As with any large-scale project, monetization is crucial for long-term sustainability. Omega Labs has several potential monetization strategies for Subnet 24 Omega. One approach could involve selling access to the dataset, allowing researchers and developers to use the data for their projects. Another possibility is allowing miners to prioritize certain types of data based on demand, creating synthetic data for specific use cases.
The decentralized nature of BitTensor opens up new opportunities for inter-subnet communication and monetization. Different subnets within the BitTensor ecosystem could exchange data, with one subnet's data benefiting another. This collaborative approach would create an ecosystem where data flows freely, benefiting all participants and reducing the risks of centralization.
Conclusion
Subnet 24 Omega represents a bold step forward in the world of AI and multimodal data collection. By leveraging BitTensor's decentralized architecture and incentivization system, Omega Labs is building the largest open-source multimodal dataset, designed to power the future of AI. With applications ranging from entertainment to education and beyond, the project has the potential to revolutionize how we build, train, and interact with AI systems.
As Omega Labs continues to expand its dataset and explore new modalities, the AI community is eager to see how Subnet 24 Omega will shape the future of AI research. Whether it's generating zero-shot multimodal content or training any-to-any models, Subnet 24 Omega is paving the way for a new era of artificial intelligence.
Source : @Opentensor Foundation