Exploring the Evolution and Mechanisms Behind OpenTensor’s Subnet Development: A Deep Dive into Emission Control and Niche Model Adaptability
OpenTensor is pushing the boundaries of decentralized AI networks, continuously refining its structure and emission control to optimize subnet efficiency. This article explores the journey behind the Detto solution, the challenges faced, and the innovations introduced to develop and manage subnets effectively. We'll also dive into the workings of Victor's Nich Net, a pioneering subnet focused on creating value through niche models and optimizing GPU usage for AI model training.
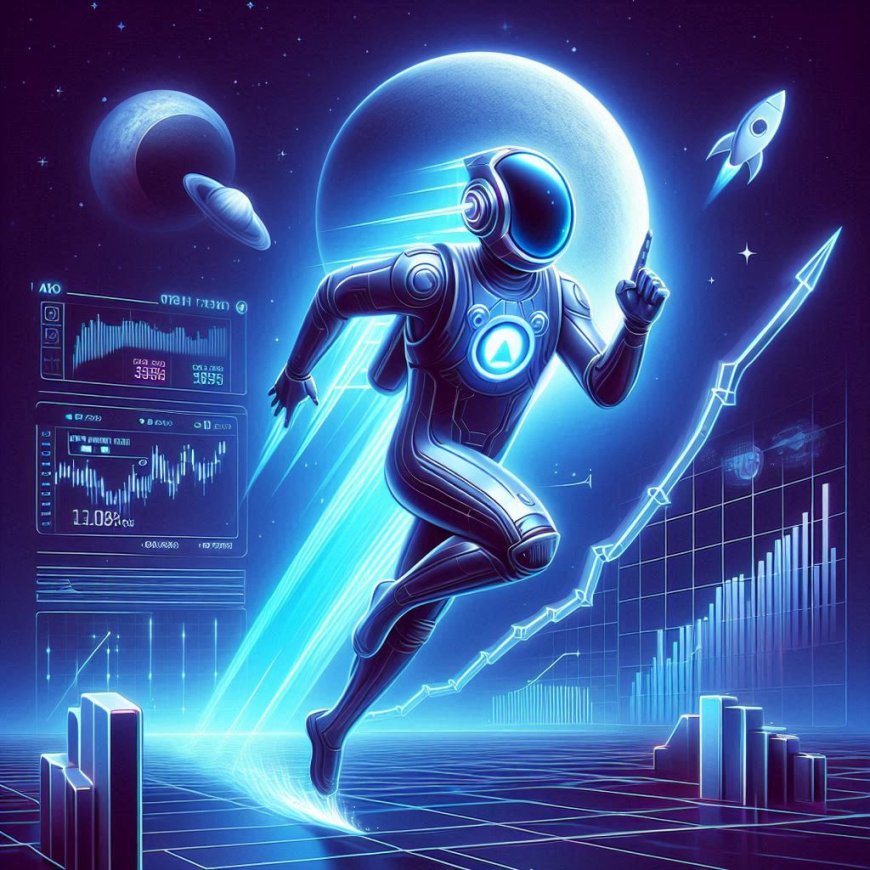
The Detto Solution and Emission Control
Back in December, OpenTensor introduced the Detto solution, a proposal that many initially struggled to comprehend. However, a deeper analysis of the sub-networks and their emission distributions reveals the necessity for DAOW—a decentralized method for emission management that isn’t tied directly to the root network.
Discussions on Twitter have highlighted various solutions, such as assigning weights to the root network to decrease emissions. As of now, approximately 7% of emissions have been reduced across the network, indicating the ongoing need to fine-tune emission levels within subnets. The incentive mechanisms in place were designed to accomplish this, but Detto offers a more market-driven approach.
This system dynamically sets emission rates based on subnet efficiency. If a subnet proves inefficient, where miners sell more than they produce, the subnet’s price adjusts accordingly, which in turn influences its emission levels. By leveraging market dynamics, Detto helps regulate inflation within the network.
Nich Net: Victor’s Vision for a Decentralized and Specialized AI Network
Victor, one of the core developers behind the network, brings us back to the early stages of Nich Net—a subnet designed to make AI universally accessible and tailored to niche needs. Originally conceived before the Revolution upgrade, Victor transitioned from mining to developing subnets, driven by a belief in the value he could add.
Victor explains the importance of creating subnets that incentivize multiple actors to participate while preventing any single miner from dominating the network. Additionally, the goal is to maximize the value for each validator by ensuring they derive benefits proportionate to their TOW (Tokens of Work) holdings.
The Concept Behind Nich Net
The name "Nich" derives from the idea of creating specialized models for different categories or niches rather than relying on a single, generalized AI model. This approach increases the overall intelligence and adaptability of the network. For instance, instead of having one model for image generation, Nich Net segments them into categories like realistic images, anime-style images, and variations focused on speed, cost, or quality.
Victor initially launched the subnet with three models, expanding it to seven, ensuring a consistent framework for development. By diversifying models and adjusting them based on demand, the subnet adapts dynamically, ensuring flexibility and optimizing resources.
Emission Control and Bandwidth Optimization
In Nich Net, emission levels are updated weekly based on demand, ensuring that all validators have equal access and encouraging fair competition among miners. The system uses both synthetic and organic requests to optimize GPU usage. After organic queries are fulfilled, the remaining capacity is allocated to synthetic requests, preventing wasted computing power.
To validate a miner’s capacity, validators test the advertised capabilities, ensuring miners deliver on their promises. The emission rate is then distributed according to each validator's share of the total TOW, ensuring dynamic load balancing between synthetic and organic demands. This approach ensures resources are distributed efficiently without favoring larger or smaller validators.
Technical Challenges and Future Updates
One major challenge is maintaining the subnet’s efficiency. Registration costs on Nich Net are currently high, with a rate of 1.12 TOW per validator. The team is working to reduce inflation by managing the subnet's burn rate. Although tests have shown positive outcomes, adjustments are ongoing to find the optimal balance between speed and volume.
Incentivizing altruistic behavior is another innovative aspect. Validators are encouraged to support subnets that positively impact the network, which may result in higher rewards. This approach fosters a collaborative environment where subnet operators are motivated to add value.
Victor discusses plans for the next update, which will include higher volume incentives to maintain decentralization and competition within the network. This will further enhance the subnet’s adaptability and capacity.
Integration and Partnerships
OpenTensor's front-end tools, such as the miner dashboard, facilitate the storage and retrieval of datasets and models. Collaboration with other subnets enables API integration for various applications, including image generation. Future expansion of model offerings will depend on demand, but the system is designed for scalability, allowing validators to create and monetize their own APIs.
The team is currently working with partners to offer API access for various applications, with future collaborations in the pipeline. Notable partnerships include collaborations with Tobot and Social Tensor, both leveraging OpenTensor’s models for their applications.
Dataset Development and Model Improvement
Victor reveals ongoing work on a new image generation model called "Proteus BT" (Big Tensor). In collaboration with partners, OpenTensor is developing a synthetic dataset to train the model, generating six million images per day. The dataset, which currently consists of several hundred thousand high-quality images, aims to train models from scratch, with filters applied to select the top 10% of images for quality.
By integrating multiple LoRAs (low-rank adaptation), the team supports a wide variety of styles using the same models, continuously improving the dataset in a feedback loop. This allows the network to evolve and support various use cases, from hyper-realistic images to anime-style graphics.
Performance Scoring and Future Roadmap
Victor explains their approach to performance scoring. For example, instead of using a linear penalty for slower processing times, the system uses a nonlinear penalty by cubing the processing time. This ensures a consistent response time, crucial for applications requiring reliability. Volume scoring adapts as the network grows, efficiently handling a broad range of volumes without constant updates.
As part of the roadmap, the team plans to push more models to production and increase usage by upgrading the API and documentation for developers. The Proteus BT model release is scheduled for May, and updates to the user interface (UI) will enhance functionality and usability.
Team Dynamics and Expansion Plans
The team behind OpenTensor consists of four core developers: Victor, William, Dong, and Tan, with machine learning backgrounds. Despite the small team size, the group plans to expand, particularly focusing on front-end and sales roles. However, Victor emphasizes the efficiency of small teams in the early stages of development, noting the plan to keep the core team compact.
Conclusion
OpenTensor is shaping the future of decentralized AI networks through innovative emission control, subnet development, and specialized model integration. The Detto solution and Nich Net are just the beginning, with more updates, partnerships, and improvements on the horizon. As the team expands and the network evolves, OpenTensor remains committed to pushing the boundaries of AI accessibility and adaptability.
Source : @Opentensor Foundation