The Evolution of Open-Source AI: How BitTensor's Subnet 6 is Revolutionizing Language Model Development
The landscape of artificial intelligence is rapidly evolving, with open-source initiatives making significant strides in catching up to proprietary giants like OpenAI. At the forefront of this revolution is BitTensor's innovative Subnet 6, a groundbreaking approach to developing and evaluating language models. This article delves into how Subnet 6 is changing the game in open-source AI development, the challenges it addresses, and its potential to reshape the future of machine learning.
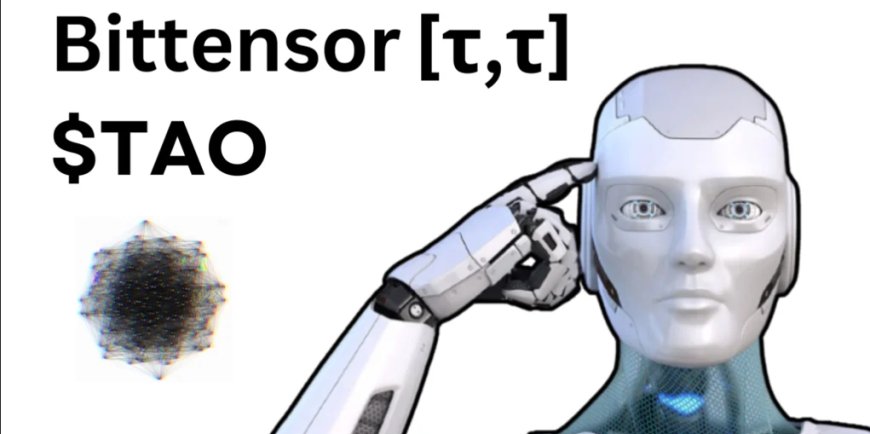
The Rise of Open-Source AI
In recent years, the open-source AI community has made remarkable progress in developing language models that rival the capabilities of GPT-3.5. This achievement is particularly impressive considering the financial constraints faced by open-source projects compared to well-funded companies like OpenAI. The key to this success lies in the community's ability to innovate and maximize performance with limited resources.
One of the most significant contributors to this progress is News Research, a community that began as a group of AI enthusiasts on Discord. Their flagship project, the New Hermes model, has become one of the most widely used open-source AI models. By porting this model to various architectures such as LLaMA, LLaMA 2, and Mistral, News Research has pushed the boundaries of what's possible with open-source AI.
The Challenge of Benchmarking and Data Contamination
As open-source models have improved, a new challenge has emerged: the reliability of benchmarks. Many researchers have focused on achieving high scores on popular benchmarks, particularly the Hugging Face Open LLM leaderboard. However, this approach has led to problems such as overfitting and data contamination.
Data contamination occurs when training data inadvertently includes information from test sets or when models are fine-tuned on benchmark data. This issue isn't always intentional; for example, it's suspected that some GSM8K data might be inadvertently included in the Mistral 7B base model. As language models become more prevalent, finding truly "clean" training data becomes increasingly difficult.
The prevalence of cheating or unintentional data contamination has led to a loss of trust in traditional leaderboards within the open-source community. Alternative evaluation methods, like the Chatbot Arena's leaderboard, have their own limitations, such as long waiting times and a limited selection of models for testing.
Enter BitTensor's Subnet 6
To address these challenges, BitTensor has developed Subnet 6, a revolutionary approach to developing and evaluating language models. Subnet 6 introduces the first continual, quantitative evaluation mechanism in the AI field. This system provides a constantly updating, cheat-proof benchmark that aligns with the goal of creating truly capable language models.
The key innovation of Subnet 6 lies in its evaluation method. Models are evaluated only on brand new data generated by Subnet 18, typically created within the last 15-20 minutes. This approach solves several problems:
- It prevents overfitting to known benchmarks, as the evaluation data is constantly new and unseen.
- It aligns the goal of model development with creating a model that performs as close to GPT-4 as possible, since the synthetic data is generated using GPT-4.
- It creates a continuously improving benchmark, as the evaluation data is always fresh and potentially becoming more complex over time.
The Power of Synthetic Data
Central to Subnet 6's approach is the use of synthetic data. While not inherently superior to human-generated data in all cases, synthetic data offers several advantages:
- Greater control over quality, consistency, and formatting
- Easier production of instruction-response pairs and other structured data formats
- Ability to ensure a baseline of quality and desired characteristics in the training data
The future of AI training may involve a combination of synthetic and human-generated data. While synthetic data can cover a wide range of tasks and ensure consistency, there will likely always be a need for some new, external data to introduce novel information and keep models up-to-date with real-world knowledge.
Incentivizing Open-Source Development
One of the most significant innovations of Subnet 6 is its incentive structure. By monetizing open-source machine learning models, Subnet 6 provides a much-needed financial incentive for researchers and developers to contribute to open-source AI projects.
This system embodies the principles of meritocracy and decentralized AI. It allows anyone, even anonymous contributors, to submit models that are judged solely on their merits. This approach has enabled previously unknown individuals to earn significant rewards while contributing to open-source development.
The incentivization built into Subnet 6 addresses a crucial issue in open-source AI development. Many open-source AI companies start with idealistic goals but eventually face roadblocks due to lack of monetization. By incorporating incentives from the beginning, Subnet 6 aims to keep models open and accessible while providing the necessary financial support for continued development and improvement.
Challenges and Future Directions
While Subnet 6 represents a significant step forward in open-source AI development, challenges remain. One of the most pressing is ensuring the right balance and diversity of tasks within large synthetic datasets. It's not just about scale, but also about the right ratios of different types of data and tasks.
Improving synthetic data generation is an ongoing process. It involves iteratively expanding the coverage of different tasks and use cases, addressing weaknesses as they are discovered, and maintaining a balance in the representation of various types of information. The goal is to create datasets that are not only large but also diverse enough to train models that can perform well across a wide range of tasks.
Looking forward, there are several areas for improvement and expansion in the BitTensor ecosystem. One suggestion is to implement atomic cross-subnet interactions, allowing for more seamless data flow between different subnets. This could eliminate the need for intermediaries like Weights & Biases in the current setup.
The Road to Surpassing GPT-4
The open-source AI community has set its sights on a lofty goal: surpassing the capabilities of GPT-4. This may be achieved through a combination of improved fine-tuning, as facilitated by Subnet 6, and advancements in pre-training from other subnets.
The potential for surpassing GPT-4 also lies in collaborative efforts like the hive mind subnet on BitTensor, which aims to combine computational resources from various participants and incentivize contributions of hardware and model improvements. By leveraging the collective compute power and expertise of the community, open-source efforts may eventually surpass the capabilities of any single organization.
Conclusion
BitTensor's Subnet 6 represents a significant leap forward in open-source AI development. By addressing the challenges of benchmarking, data contamination, and incentivization, it paves the way for the creation of more powerful and reliable language models.
The use of synthetic data, continual evaluation, and innovative incentive structures positions the open-source community to compete with and potentially surpass proprietary models. As the ecosystem continues to evolve, we can expect to see even more groundbreaking developments in the field of AI.
For those interested in contributing to these efforts, studying existing high-quality datasets, such as the one behind Open Hermes 2.5, can provide valuable insights into creating diverse and effective training data. The open-source AI community continues to push boundaries, driven by innovation and collaborative efforts, aiming to create models that can compete with and potentially surpass proprietary alternatives.
As we look to the future, it's clear that the open-source AI community, empowered by innovations like BitTensor's Subnet 6, will play a crucial role in shaping the future of artificial intelligence. By fostering collaboration, incentivizing contributions, and continuously pushing the boundaries of what's possible, open-source AI is poised to democratize access to advanced language models and accelerate the pace of innovation in the field.
Source : @The Bittensor Hub.